We investigate the problem of choice overload – the difficulty of making a decision when faced with many options – when displaying related-article recommendations in digital libraries. So far, research regarding to how many items should be displayed has mostly been done in the fields of media recommendations and search engines. We analyze the number of recommendations in current digital libraries. When browsing fullscreen with a laptop or desktop PC, all display a fixed number of recommendations. 72% display three, four, or five recommendations, none display more than ten. We provide results from an empirical evaluation conducted with GESIS’ digital library Sowiport, with recommendations delivered by recommendations-as-a-service provider Mr. DLib. We use click-through rate as a measure of recommendation effectiveness based on 3.4 million delivered recommendations. Our results show lower click-through rates for higher numbers of recommendations and twice as many clicked recommendations when displaying ten instead of one related-articles. Our results indicate that users might quickly feel overloaded by choice.
We presented this work as a paper at the BIR Workshop 2017 (Bibliometric-enhanced Information Retrieval) workshop (co-located with ECIR 2017, European Conference on Information Retrieval). You can find the full paper here (PDF)
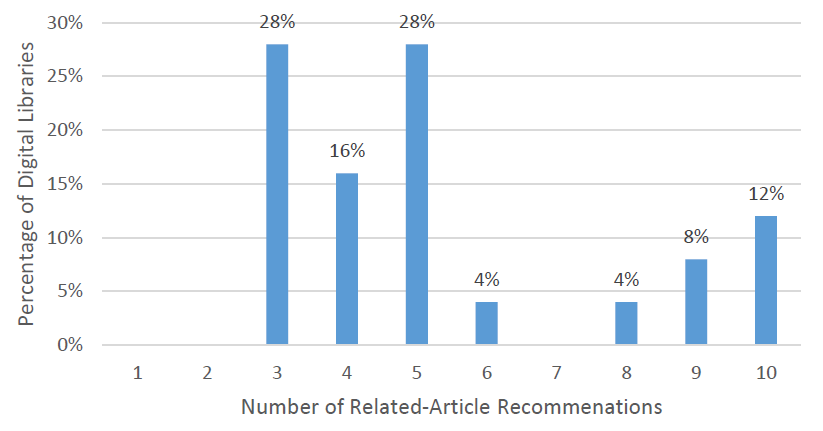
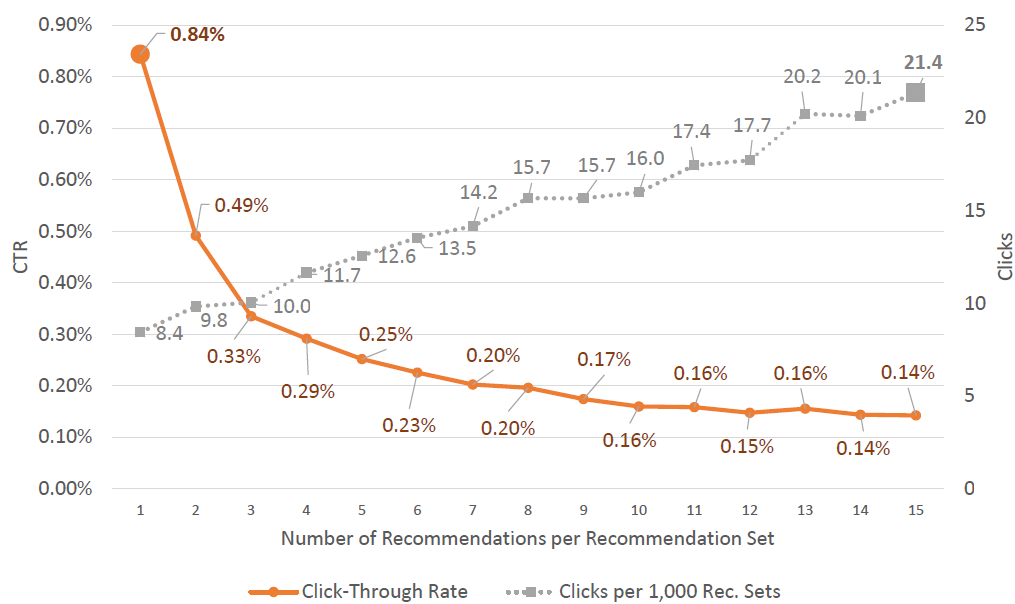